7 steps to building a data foundation for organizational design
Seven iterative steps to good quality data
Published by Orgvue
Home > Resources > article > 7 steps to building a data foundation for organizational design
Organizational data is often incomplete, spread across numerous systems, or hidden in spreadsheets. While no dataset will ever be perfect, building a reliable data foundation will bring you closer to business-changing insights on a continuing basis. In this article, we look at seven iterative steps to collecting, cleaning, and integrating data for organizational design, as well as visualizing and analyzing it.
Step 1: Follow your intuition and get the focus right
For many years, strategy consultants have used hypotheses to focus their research – and it works. Data and analytics are not just about facts and figures. Often the best insights come from intuition, investigation, and creative thinking. When building a data foundation, it pays to think beyond simple organizational metrics such as headcount and absence. Go back to your design criteria to direct your focus.
When doing a major transformation, there are certain metrics you’ll almost certainly need. But remember, assembling your baseline data is not a one-off but an ongoing task that should support the continuous development of your organization.
Step 2: Make the data collection process valuable for data owners
As a rule, people don’t like to part with data, so be sure to explain why you’re collecting it, how it’s going to help, and what the consequences are of not getting it.
First and foremost, you have to answer the question ‘what’s in it for the data owners if they hand over their data? You have to demonstrate the value they’ll be contributing or getting back by explaining the end-to-end process the data will go through and the benefits it will deliver.
One approach is to gamify the process. To give a real-life example, to encourage a network of hospitals to clean their data, the organizing project team created a public league table. Before this, all hospitals had made very little progress. But within a week of the league table being posted, progress improved exponentially. By gamifying it, the project became team effort.
Step 3: Locate and pull together existing data
Before you jump straight into collating new data, consider your existing data. Often you already have much of the data you need to see the’ as-is’ picture of your organization. You just need to bring it together. Doing the following will help you:
- show those working hard to make data available how the information is being used
- start to answer your hypotheses and make sure you’re collecting the right data
- gain a much better understanding of data cleaning and collection process.
Once you know what data you need, the next step is to determine where to source it. In doing this, you need to understand:
- what systems are being used and where in the organization. Often different geographical locations will use different systems.
- how the different data types you need map to these systems and which types don’t exist currently
- who owns which system and what the process is for extracting the data.
Step 4: Visualize your data
Visual representation is a key part of the data analytics process. When visualizing your data, try to stay away from spreadsheets and use software specifically designed to bring your data to life and achieve buy-in from the people working with it.
Data visualization can also uncover some basic insights that will help reaffirm your hypotheses and ensure you’re going in the right direction. When looking for these insights, think through the various visualization options:
- traditional statistical representations – bars, columns, lines, scatter plots, box plots, Gantt charts, tables, dashboards
- additional analytical elements such as colouring, scaling, and hierarchies
- plotting a fourth dimension of time to highlight trends.
Visualizing data will add value at each stage of building your data foundation. For example, looking at data quality can help identify gaps and show you where there may be inaccuracies ( see our data types and patterns dashboard below).
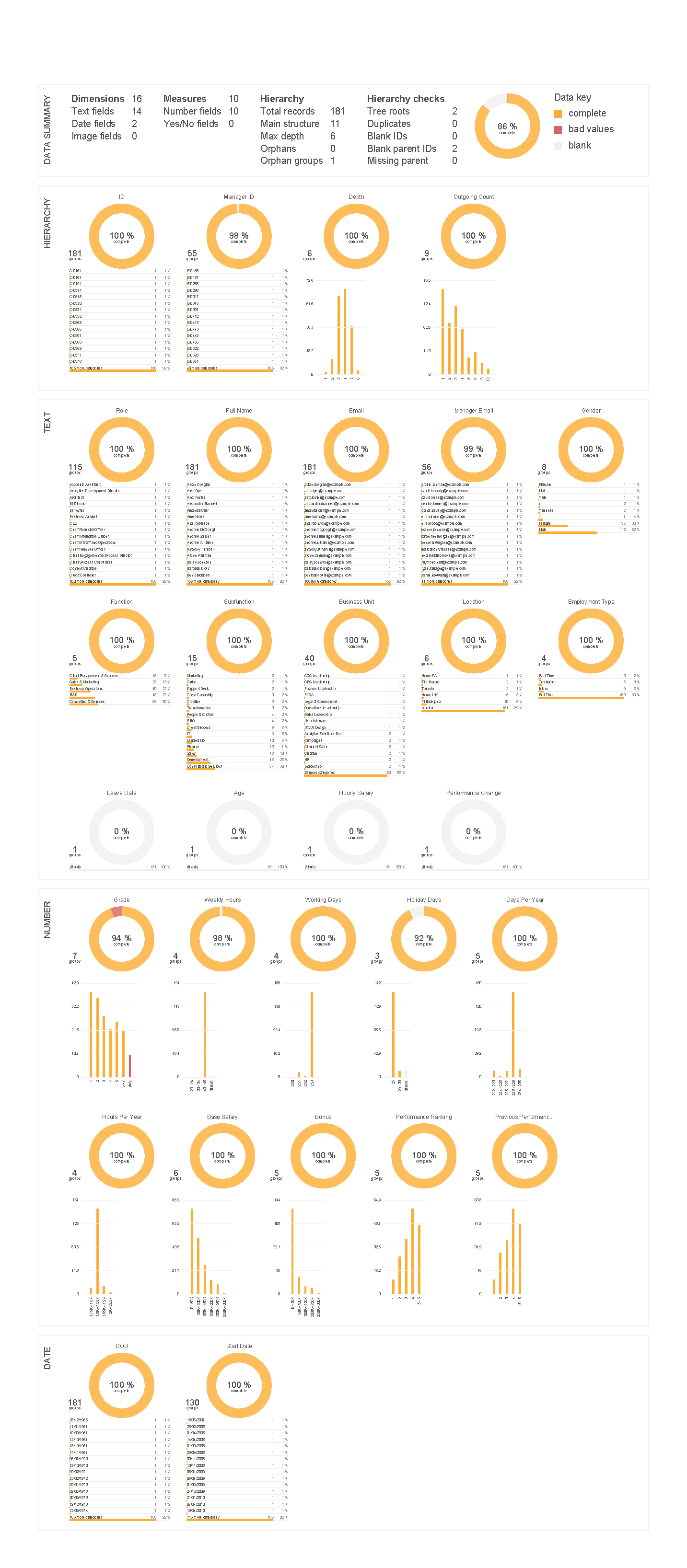
Step 5: Create rules and clean data centrally
Whenever you bring data together, there will be data quality issues. So, it pays to create clear rules on data structure from the outset. And once your data is clean, you need to steps to keep it that way. Take gender as an example. Male employees may be tagged as male, m, 0 or 1, MALE, so you should be clear and consistent in which tag you use.
Step 6: Crowdsource your data
Make it easy for people to supply additional data elements that will further improve quality. Big data is all very glamorous, but the most value often comes from getting the basics right. Here are three tips to help you when crowdsourcing data:
- Make data easy to upload. If the upload process is complex, people will not bother. Sending out a webform that takes two minutes to complete will encourage instant updates.
- Report data back to owners. Those who know the data best should be the ones who interrogate it. They will get new insights from the process. They are the ones who will produce new insights, identify outliers, and uncover data quality issues.
- Make the process mobile. Often the best time to spend a couple of minutes uploading or analyzing data is when you’re in transit. If data is available on all devices, it makes it easier to review and add insight on the fly.
Step 7: Stop analyzing and start playing with your data
Once you’ve established a baseline, start exploring your data. Slice it using different dimensions and measures, and dice it using aggregators such as sum, standard deviation, and percentile. You’ll be amazed what you find.
To make better sense of your data, try breaking down insights into topics by area or theme. For example, by function, whether it’s HR, Finance, or Procurement, or by focus such as strategic, operational, or transactional. But when analyzing and drawing your insights, be careful. What might seem obvious in the data may be wrong statistically. It’s too easy to oversimplify and make errors of judgement. Correlation is not the same as causation.
Read next…
It goes without saying that data is the lifeblood of analytics. Yet many organizations still struggle to aggregate data from disparate sources and maintain the quality of that data. This article looks at the mindset change that’s needed to embed a positive data culture in your HR team and build an effective people analytics capability.
Learn more about building a healthy data culture for effective people analytics
It goes without saying that data is the lifeblood of analytics. Yet many organizations still struggle to aggregate data from disparate sources and maintain the quality of that data. This next article looks at the mindset change that’s needed to embed a positive data culture in your HR team and build an effective people analytics capability.