The cost and challenges of dirty data in business transformation
The importance of data cleansing, why dirty data costs, why dirty data happens, and the core principles of data cleaning.
Why is data cleansing important?
Dirty data can engender serious problems, costing your business time, money, and huge risks in decision-making. As the old adage goes, “garbage in, garbage out.” Still, data quality issues are often ignored in the context of organisational transformations.
Many times I saw first-hand with clients how data cleanliness was the major stumbling block to the success of their transformation projects. If you can’t get your data clean and accurate from the start, fixing it later can absorb 50% of the effort in a 6 month project. And more importantly, it can stop the project moving forward quickly enough to deliver results.
Dirty data is costing you: three challenges in addressing data quality issues
In a recent HR executives’ workshop we ran on data cleansing, our participants – thank you Gaurav Gupta, Henk Langeveld, Boudewijn van Straaten, and Marco Kippers – challenged us with some excellent questions. Do Volvo made a big deal of how clean their factories are, to sell us more cars? No! Do Amazon tell us how well they manage their website source code to encourage us to buy more books? Surely not!
Clean factories and well-managed code are the minimum requirements for making cars and e-commerce these days. And that’s also the story for business transformation. Clean datasets are minimum table stakes. To transform any business, you need to get the data under control, and keep it clean enough to answer basic questions fast to deliver value.
- How many people do we have? How many contractors? How many close to retirement?
- What skills do we have?
- What is our work now – and what will our future work be?
- How re-trainable are our staff?
So the L’Oreal question: is it worth it? Definitely. Cleaning data quickly means stopping political disputes over definitions and focusing on adding value. You deliver better decisions – better organisation design scenarios tested, better choices made, better people and key skills retained.
The participants came to a consensus that there are three key things that HR need to get right to overcome the data quality challenge:
1 – Having the right mind-set about data
Our participants argued that the issue for most HR people was not the technical challenge of data cleaning but rather the mind-set gap that it posed: does HR really believe that data can be effective? Does HR really want to gather data and test its own assumptions? Take values. As one participant argued: “People say ‘if employees meet our values they will be successful.’ But the link is just gut feeling. There is no science behind it at all!”
2 – Building a long-term Data Strategy, not just reactive data cleaning
Data cleaning, our panel pointed out, might be needed as a temporary fix when a business transforms. The business should however be thinking about the long-term approach with data prioritisation and data maintenance key integral parts of the data strategy, to avoid gradually declining quality and a cycle of repetition. And think about the added value data can bring to your day-to-day operations
3 – Seeing people data as a corporate asset
One participant in the session commented, “Your People Dataset is a resource to be maintained, developed and used just like any other corporate asset.” Just like customer or research data, people data can bring a wealth of valuable business insights such as:
- What investments you have made in your people
- Who you recruited and why
- Who chose not to join you at one point in time and why
- What worked in organisation structures
- What did not work
So what causes dirty data?
We were agreed as a group that indiscipline or deliberate ill-will towards the HR systems were not fundamental causes of dirty data. So what’s the issue? The issue is that HR data is intermittently changing in a continuously changing world. Many different details move in many small ways: responsibilities, grades, reporting lines, even job titles. Those details are not always updated centrally. The more the world moves towards Agile organisational principles, the more the facts of organisational life will move fluidly from the original hierarchical design, and the harder it will be to keep People Data up to date. The types of data issues might include:
- Multiple systems, new systems
- Data gaps
- Positions vs People data
- Org Structures & Vacancies
- Responsibility mapping
- Project mapping
- Agile organisations
What are the consequences of dirty data?
The group were convinced that dirty data genuinely did have an impact, especially at the start of any kind of transformation programme:
- “It slows you down.”
- “You make wrong decisions on where to base your operations. Who to hire. Who to keep.”
- “It inhibits your ability to speak at the Executive level”
- “You can’t cut through the myths”
- “People haggle over data accuracy to avoid talking about the real issues.”
What are the core principles of data cleaning?
So the group discussed and settled on some key principles for cleaning data:
- Prioritise your data – what is really needed?
- One source of the truth – agree the source of each variable
- Get it clean and keep it clean – only do data cleaning if part of the wider data strategy
- Clear ownership. E.g.: ‘employees are responsible for their own data’
- Publish your data definitions
- Controlled cleaning: if you clean, be able to audit & track back
- Intelligent cleaning: learn from the cleaning changes made in the dataset
- Publish your data principles!
Comparison with the Orgvue approach
We found the discussion of dirty data useful to compare with what we usually see in transformation work. Our approach is that data has to be cleaned in the first month. We believe that data accuracy has to be owned as locally as possible, and fed back to data owners in charts and completeness rankings as fast as possible. Our typical plan is to get all org charts and hierarchical data clean in Week 1, and to get all other key data collected in Weeks 2-4. That is what allows any transformation programme to get started and to build on the basis of the facts.
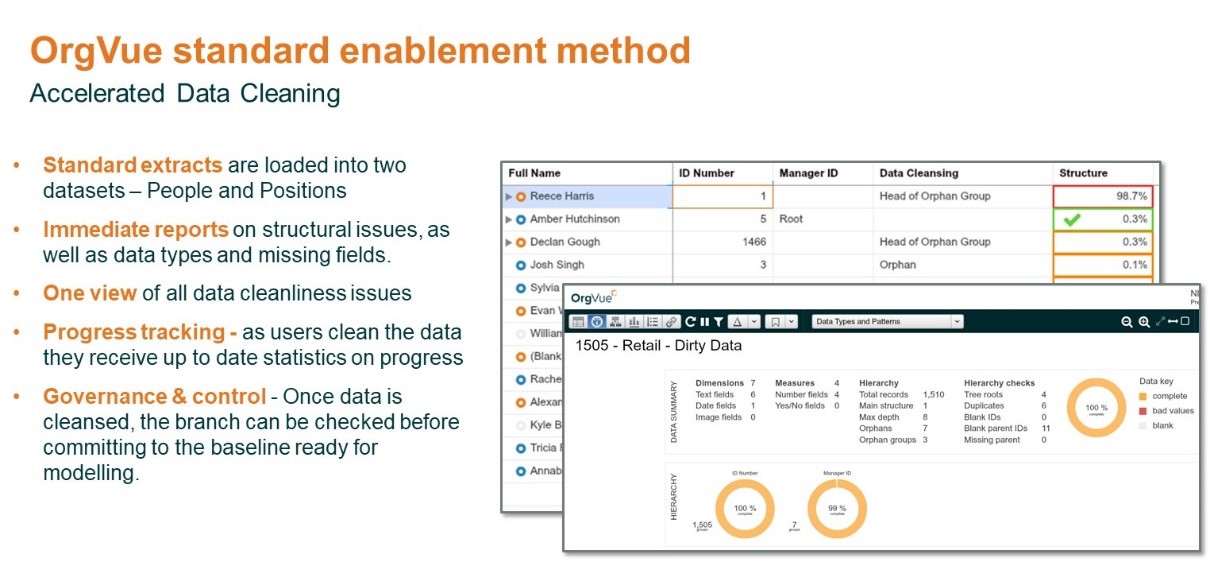